Generative AI in Cyber Security Market Growing at a CAGR of 22.1% Driven by Improvements in Product Design
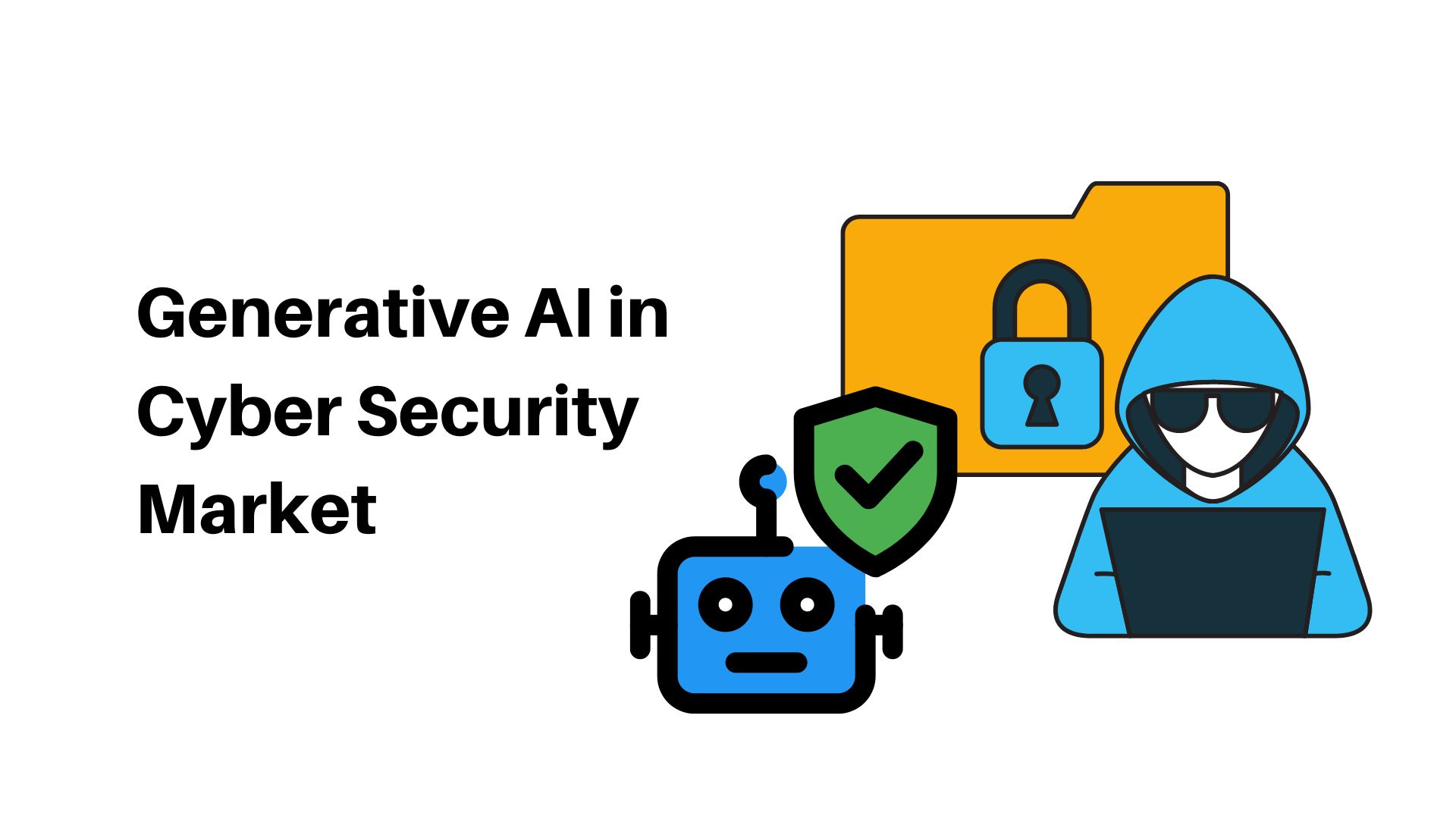
Page Contents
Market Overview
Published Via 11Press : The Generative AI in Cyber Security Market size is expected to reach USD 11.2 Bn by 2032, up from its current value of USD 1.6 Bn in 2022, growing at an annual compound growth rate (CAGR) of 22.1% from 2023-2032.
Generative AI, a subset of artificial intelligence, has become an invaluable asset in cybersecurity. Generative AI utilizes algorithms and models to generate unique content such as images, text or even code – in this instance generating AI is being leveraged as an innovative solution against ever-evolving cyber threats.
Traditional cybersecurity methods typically rely on static defenses with predefined rules and patterns to detect and mitigate attacks, yet modern cybercriminals have become more adept at exploiting those weaknesses than ever. Generative AI offers an alternative by continuously learning from vast amounts of data while responding proactively to emerging threats.
By employing generative AI, cybersecurity professionals can extend their capabilities in various areas. One such application of this powerful tool is in the detection of previously unknown malware and zero-day attacks, as Generative AI algorithms can analyze patterns in data to detect anomalous behavior that could signal potential danger. By taking such an aggressive stance against potential attacks before they cause significant damage, organizations are better able to spot and stop attacks before any serious harm comes their way.
Generational AI has also proven useful in automating threat response. By training AI models on historical data and cybersecurity best practices, organizations are creating algorithms capable of autonomously responding to attacks in real-time – without needing human intervention – identifying attack patterns, isolating infected systems, and applying remediation measures without delay or risk of human error. This helps speed response time as well as reduce human errors. Generative AI plays an essential part in strengthening cybersecurity analyst capabilities. By automating routine tasks such as data analysis, log monitoring and vulnerability scanning, analysts can focus on more strategic aspects of their work – this allows more efficient use of resources while helping tackle emerging threats more effectively.
Request Sample Copy of Generative AI in Cyber Security Market Report at: https://marketresearch.biz/report/generative-ai-in-cyber-security-market/request-sample/
Key Takeaways
- Generative AI (GenAI) is a form of artificial intelligence (AI), employing algorithms and models to generate new and original content.
- GenAI has become increasingly important for cybersecurity by being used to detect and respond more quickly to emerging threats.
- Generative AI can aid organizations in the detection and elimination of previously unknown malware and zero-day attacks by analyzing patterns in data.
- Furthermore, Generative AI enables automated threat response services enabling organizations to autonomously mitigate attacks in real-time.
- By automating routine tasks such as data analysis and vulnerability scanning, generative AI strengthens cybersecurity analysts.
- Its deployment requires access to large amounts of high-quality data for training purposes as well as ongoing monitoring to ensure security and reliability.
- Generative AI offers opportunities for cybersecurity in the future, including enhanced threat detection and prevention capabilities.
- While data privacy concerns and adversarial attacks pose threats against critical assets, generative AI holds promise as an effective method to guard them.
Regional Snapshot
- North America:
North America is leading the charge when it comes to adopting generative AI for cybersecurity purposes, boasting both advanced technological infrastructure and an abundance of cybersecurity companies that employ it. Major US-based players such as IBM, Palo Alto Networks and FireEye have made substantial investments into research and development of this form of artificial intelligence research; their market primarily utilizes this form of artificial intelligence for threat detection, automated response and security analytics purposes. - Europe:
Europe has experienced significant developments in generative AI for cybersecurity applications. Countries such as Great Britain, Germany and France are actively investigating how generative AI technologies can be integrated into their cybersecurity frameworks; European organizations prioritize using it for real-time threat intelligence gathering, anomaly detection and vulnerability assessments. GDPR regulations have furthered responsible use by emphasizing data privacy and security. - Asia-Pacific:
The Asia-Pacific region shows immense promise for using generative AI in cybersecurity applications. Countries like China, Japan and South Korea are investing heavily in AI research and development efforts that target cybersecurity applications; specifically network security, intelligent threat hunting and predictive analytics using this form of artificial intelligence (AI). Collaborations among governments, academia and industry players drive innovation while supporting growth of generative AI as part of cybersecurity practices. - Latin America:
Latin America has slowly begun adopting generative AI for cybersecurity applications, though at a much slower rate compared with other regions. Countries such as Brazil and Mexico are showing increased interest in using AI technologies to bolster their cybersecurity defenses. Generic AI applications in Latin America mainly include incident response, malware analysis, security operations optimization as well as incident management functions; collaborative efforts between public and private sectors are crucial for further progress of adoption of this type of technology. - Middle East and Africa:
Both regions are making steady strides in applying generative AI to cybersecurity applications. Countries like Israel and the United Arab Emirates have emerged as hubs of cybersecurity research and innovation, employing it for threat intelligence sharing, deception technologies and advanced malware detection using Generative AI systems. However, challenges related to data availability, infrastructure requirements and regulatory frameworks need to be overcome for wider adoption of such AI technologies.
Any inquiry, Speak to our expert at: https://marketresearch.biz/report/generative-ai-in-cyber-security-market/#inquiry
Drivers
- Cyber Threats Are Proliferating Rapidly:
Cyber threats have evolved at an incredible pace and traditional security measures struggle to keep up with them. Generative AI provides a proactive and dynamic solution that detects and responds rapidly to new attacks by providing better defense against sophisticated attacks. - Need for Real-Time Response:
In response to advanced threats, organizations require real-time response capabilities in order to minimize their effects on organizations. Generative AI allows automated threat detection and response reducing response times between an attack being identified and its mitigation – which are critical components for mitigating damage or loss caused by attacks. - Growing Volume and Complexity of Data:
With so much digital information being generated by interconnected devices, cybersecurity analysts face immense amounts of data that is difficult to manually process and interpret manually. Generative AI excels at processing large datasets efficiently while extracting meaningful insights while also recognizing patterns that could indicate malicious activity. - Shortage of Trained Cybersecurity Professionals:
Generative AI offers an effective solution to address the shortage of skilled cybersecurity professionals worldwide. By automating routine tasks such as log monitoring and vulnerability scanning, analysts can focus on more complex aspects of cybersecurity rather than mundane, mundane routine tasks – this augmentation of human capabilities enhances overall efficiency and effectiveness when combatting cyber threats. - Industries and Regulatory Compliance Requirements:
Finance, healthcare and government industries face stringent regulatory compliance requirements to safeguard sensitive data. Generative AI assists these industries by improving threat detection, data privacy management and incident response capabilities to help meet compliance obligations while upholding integrity and security in systems and data storage systems. - Technological Advancements:
Rapid advances in computing power, data storage capacity and algorithm development have dramatically advanced generative AI models' use in cybersecurity applications. Deep learning algorithms, neural networks and natural language processing techniques have significantly enhanced their accuracy and capabilities for cyber threat detection and response purposes.
Restraints
- Data Privacy and Security Concerns:
Generative AI algorithms require vast quantities of high-quality data for their training, raising significant concerns over privacy and security. In order to protect their sensitive information from unauthorized access and misuse, organizations must take steps such as anonymization, encryption, and comply with data protection regulations in order to keep it private and safe. - Lack of Quality Training Data:
Generative AI models' success depends on having access to high-quality training data. Acquiring this kind of data in cybersecurity, however, can be extremely challenging due to its sensitivity and confidentiality requirements. Furthermore, lacking labeled data for specific cyber threats may impede their development and accuracy as generative AI models. - Adversarial Attacks and Bias:
Generative AI models are vulnerable to adversarial attacks from malicious actors that deliberately manipulate input data in order to deceive or disrupt the model's functioning, diminishing both effectiveness and reliability in terms of cybersecurity. Furthermore, biases present in training data can produce inaccurate or discriminatory outcomes with unintended repercussions that are potentially unintended consequences of such misuse. - Interpretability and Explainability:
Generative AI models often act like black boxes, making it hard for humans to comprehend or explain their decision-making processes. Lack of interpretability and explainability undermines trust and accountability within cybersecurity environments, so organizations and regulatory bodies need to establish guidelines and frameworks for auditing and explaining decisions made by generative AI systems. - Scalability and Resource Requirements:
Generative AI models can be extremely computationally intensive and may require significant computing resources and infrastructure for training and deployment. Small and midsized organizations may face difficulty scaling these solutions due to limited scalability or resource availability issues; this may hinder their adoption of such AI solutions. - Ethics and Legal Considerations:
Generative AI use in cybersecurity raises a range of ethical and legal considerations. Issues such as algorithmic bias, unintended consequences and liability associated with automated decisions must be considered when using such technologies responsibly and ethically; regulatory frameworks or ethical guidelines may need to be developed as a result.
Opportunities
- Proactive Threat Detection: mes Generative AI provides proactive threat detection by analyzing large volumes of data, recognizing patterns and anomalies that might indicate cyber attacks. By continuously learning from new threats, generative AI models can detect previously unknown malware and zero-day vulnerabilities – providing early warnings and lessening their impact.
- Automated Incident Response: Generative AI provides organizations with the capability to respond swiftly and proactively to cyber incidents by automating response. Leveraging historical data, these models can autonomously analyze and respond to security incidents quickly by isolating compromised systems, applying remediation measures quickly, and mitigating threats rapidly.
- Augmented Cybersecurity Analysts: Generative AI technology augments the capabilities of cybersecurity analysts by automating repetitive and mundane tasks that free them up for more complex and strategic activities such as threat hunting, strategic planning and policy creation. Working hand in hand, both AI and human analysts improve overall cybersecurity posture and effectiveness.
- Enhanced Decision-Making: Generative AI offers cybersecurity professionals improved decision-making capabilities by processing vast amounts of data to detect vulnerabilities and risks that exist within it, giving decision-makers actionable insights and recommendations regarding resource allocation, risk mitigation strategies and security investments.
- Security Analytics and Intelligence: Generative AI allows organizations to harness the power of big data analytics to gain meaningful insights from complex security-related datasets. When combined with other analytic techniques, organizations gain visibility into their entire cybersecurity environment for improved threat intelligence, incident response, and vulnerability management.
- Adaptive Security Measures: Generative AI models can adapt and evolve in response to new information and changing threat landscapes, learning from data to stay ahead of emerging threats and updating algorithms/models accordingly. This adaptability enables organizations to build dynamic yet resilient cybersecurity defenses capable of quickly adapting to ever-evolving attack techniques.
- Industry Collaboration and Knowledge Sharing: Generative AI's adoption in cybersecurity promotes collaboration and knowledge-sharing between organizations, industry sectors, and academia. Sharing insights, best practices, and threat intelligence allows collective defense against cyber attacks to form. Collaborative efforts result in the development of robust generative AI models which benefit everyone within this community.
Take a look at the PDF sample of this report: https://marketresearch.biz/report/generative-ai-in-cyber-security-market/request-sample/
Challenges
- Data Quality and Availability:
Generative AI models require large volumes of high-quality data for training purposes, which poses challenges in cybersecurity due to privacy concerns and limited labeled datasets. A lack of diverse and representative datasets reduces accuracy and effectiveness. - Adversarial Attacks and Model Vulnerabilities:
Generative AI models can be vulnerable to adversarial attacks from malicious actors looking to take advantage of vulnerabilities within them by manipulating input data to deceive or take advantage of any flaws within them. Such attacks could potentially result in false positives/negatives and compromise the reliability and trustworthiness of AI solutions. - Ethical and Legal Implications of Generative AI for Cybersecurity:
Generative AI's use in cybersecurity raises significant ethical and legal implications, including algorithmic bias, privacy violations, and accountability for automated decisions made by computers. Ensuring fairness, transparency, and compliance with applicable regulations and ethical guidelines must also be carefully considered when employing this form of artificial intelligence in security applications. - Interpretability and Explainability:
Generative AI models often operate like black boxes, making it challenging to interpret their decision-making processes and explain their outputs. Without sufficient interpretability and explainability, trust, accountability, and regulatory compliance suffer as a result of lack of trustworthiness and regulatory compliance issues. As such, efforts should be undertaken in developing techniques and frameworks for understanding generative AI models more clearly. - Resource Intensity:
Training and deploying generative AI models can be computationally intensive, necessitating significant computing power and storage resources. Small to mid-sized organizations may experience challenges related to resource availability, scalability and affordability when trying to deploy such AI solutions. - Skill and Expertise Gap:
For effective adoption and implementation of generative AI in cybersecurity applications, professional skill sets such as those needed to create, deploy and maintain models are necessary. However, with so few cybersecurity professionals equipped to leverage this technology efficiently. organizations face a formidable obstacle. - Compliance and Data Privacy:
Organizations using generative AI for cybersecurity purposes must abide by data protection regulations and privacy laws when using these models to store or process sensitive data. Generative AI models must adhere to legal and ethical standards when managing and processing sensitive information.
Market Segmentation
Based on Type
- Threat Detection and Analysis
- Adversarial Defense
- Insider Threat Detection
- Network Security
- Other Types
Based on Technology
- Generative Adversarial Networks (GANs)
- Variational Autoencoders (VAEs)
- Reinforcement Learning (RL)
- Deep Neural Networks (DNNs)
- Natural Language Processing (NLP)
- Other Technologies
Based on End-User
- Banking, Financial Services, and Insurance (BFSI)
- Healthcare and Life Sciences
- Government and Defense
- Retail and E-commerce
- Manufacturing and Industrial
- IT and Telecommunications
- Energy and Utilities
- Other End-Users
Key Players
- OpenAI
- IBM Corp.
- NVIDIA Corporation
- Broadcom Inc.
- Darktrace
- Cylance
- McAfee Corp.
- FireEye
- Other Key players
Report Scope
Report Attribute | Details |
Generative AI in Cyber Security Market size value in 2022 | USD 1.6 Bn |
Revenue forecast by 2032 | USD 11.2 Bn |
Growth Rate | CAGR Of 22.1% |
Regions Covered | North America, Europe, Asia Pacific, Latin America, and Middle East & Africa, and Rest of the World |
Historical Years | 2017-2022 |
Base Year | 2022 |
Estimated Year | 2023 |
Short-Term Projection Year | 2028 |
Long-Term Projected Year | 2032 |
Recent Developments
- 2021: In January, Google announced it would launch Chronicle Detect, a generative AI-powered threat detection system.
- 2022: In February, Palo Alto Networks recently announced the acquisition of Expanse, a generative AI startup. Expanse uses this method to map global attack surfaces and detect threats.
- 2023: In March, Check Point Software Technologies unveiled Quantum Armor, an AI-powered vulnerability assessment and remediation solution.
Key Questions
Q: What is Generative AI in Cybersecurity?
A: Generative AI refers to using algorithms and models to generate original content within cybersecurity. Generative AI allows organizations to quickly detect emerging threats by learning from data analysis and producing solutions proactively in response.
Q: How can Generative AI enhance threat detection in cybersecurity?
A: Generative AI performs data analysis to detect anomalies that might indicate potential threats, including previously undiscovered malware and zero-day attacks that might require proactive steps for mitigating risks. By doing this, Generative AI allows organizations to take measures early and mitigate potential issues effectively.
Q: How is Generative AI Helping Secure Cyberspace?
A: Generative AI presents opportunities in cybersecurity such as proactive threat detection, automated incident response, enhanced decision-making capabilities and advanced security analytics, along with intelligence sharing between organizations.
Q: What are the challenges associated with generative AI implementation in cybersecurity?
A: Challenges include data privacy and security concerns, lack of quality training data, vulnerability to adversarial attacks, interpretability/explainability issues, resource intensiveness as well as ethical and legal implications.
Q: Have there been any developments by companies regarding generative AI for cybersecurity purposes?
A: Companies like OpenAI, Darktrace, Cylance, Deep Instinct and Symantec have made important strides by developing AI-powered platforms and models to enhance threat detection, response and prevention capabilities.
Q: How can generative AI address the lack of qualified cybersecurity professionals?
A: By automating routine tasks, generative AI allows cybersecurity analysts to focus on more complex and strategic activities aimed at combatting cyber threats more efficiently and effectively.
Q: What regulations apply to Generative AI security considerations?
A: Generative AI used in cybersecurity must comply with data protection and privacy laws. Organizations must take care to use generative AI responsibly and ethically while adhering to all legal requirements when handling sensitive data.
Q: What are the primary drivers behind the rise in popularity of Generative Artificial Intelligence for cyber security applications?
A: Cyber threats have grown increasingly complex over the years and require immediate responses in real time, data volumes have continued to expand and complexity increase, skilled cybersecurity personnel remain scarce, compliance requirements need to be fulfilled by industries and regulators are tightening, technological developments have progressed rapidly, as has awareness about AI's potential applications – all factors driving its adoption by businesses. Generative AI in Cyber Security Market.
Contact us
Contact Person: Mr. Lawrence John
Marketresearch.Biz (Powered By Prudour Pvt. Ltd.)
Tel: +1 (347) 796-4335
Send Email: [email protected]
Content has been published via 11press. for more details please contact at [email protected]

The team behind market.us, marketresearch.biz, market.biz and more. Our purpose is to keep our customers ahead of the game with regard to the markets. They may fluctuate up or down, but we will help you to stay ahead of the curve in these market fluctuations. Our consistent growth and ability to deliver in-depth analyses and market insight has engaged genuine market players. They have faith in us to offer the data and information they require to make balanced and decisive marketing decisions.