Generative AI in Agriculture Market to Reach USD 1083.9 Mn with 24.8% CAGR In 2032
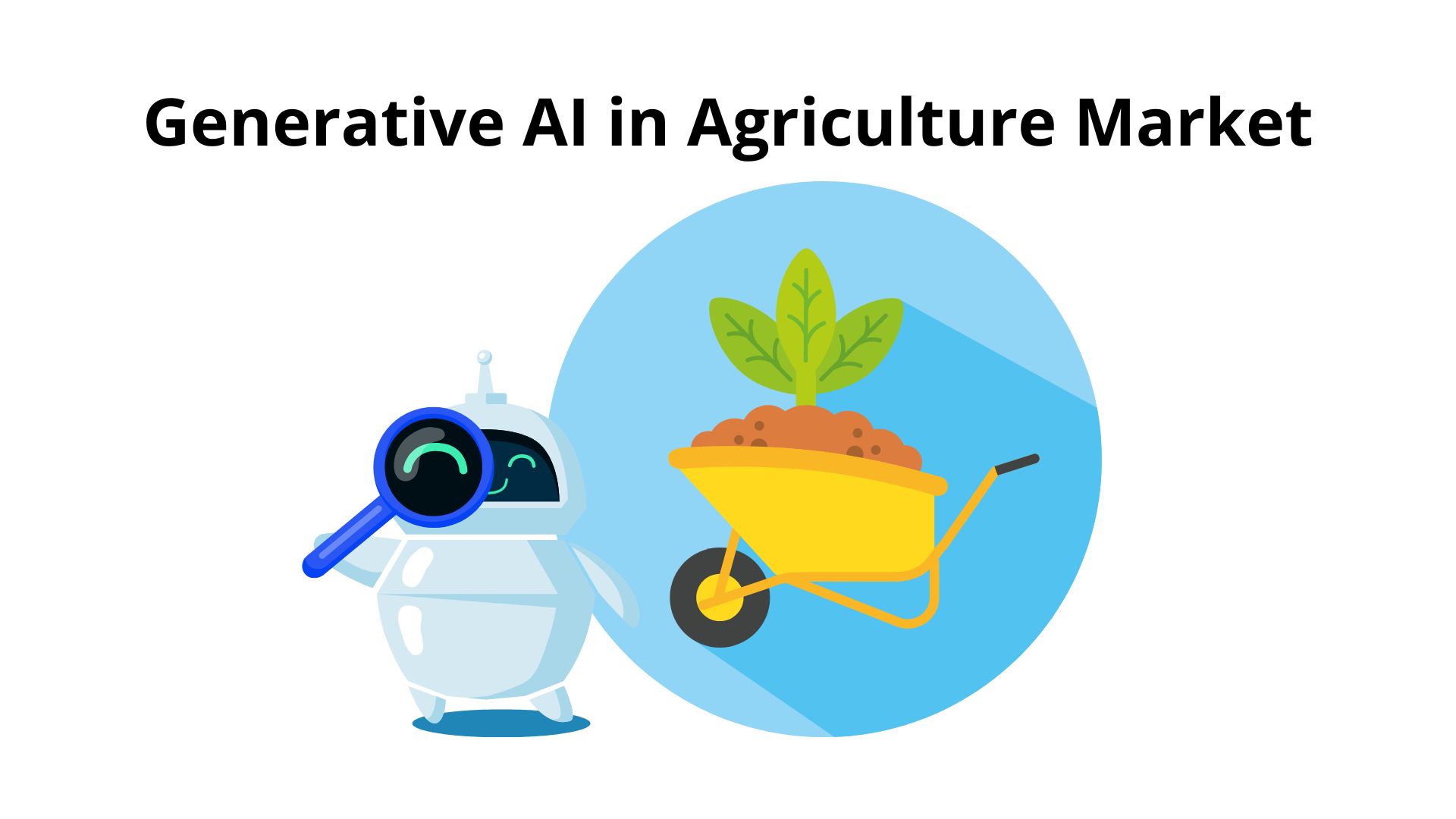
Page Contents
Market Overview
Published Via 11Press : Generative AI in Agriculture Market size is expected to be worth around USD 1083.9 Mn by 2032 from USD 125 Mn in 2022, growing at a CAGR of 24.8% during the forecast period from 2022 to 2032.
Generative Artificial Intelligence (AI) in agriculture has seen rapid adoption. Generative AI refers to using algorithms and models to generate new content based on existing data inputs; when applied in farming it has the power to transform various aspects from crop optimization to livestock management.
One key area where generative AI is making an impactful contribution in agriculture is crop management. By analyzing vast amounts of data such as soil conditions, weather patterns and yields from past harvests, generative AI models are providing farmers with insights and recommendations that assist with making informed decisions regarding planting times, fertilizer application rates and irrigation schedules – leading to increased crop yields while optimizing resource use efficiency.
Livestock management can also benefit from generative AI. Utilizing machine learning algorithms, farmers can more effectively monitor animal behavior, health, and wellbeing more effectively. Generative AI models can analyze sensor data collected via wearable devices on the animals such as accelerometers or temperature sensors in order to detect patterns or anomalies; this allows early disease detection, optimized feed optimization strategies, and overall improved welfare for animals.
Precision agriculture – which involves applying inputs based on site-specific conditions – can be greatly enhanced by using generative AI models. By gathering information from satellites, drones, and ground sensors, these AI models create detailed maps of fields with variations in soil composition, moisture levels and crop health; using this knowledge farmers can maximize distribution of fertilizers, pesticides and water while decreasing waste and environmental impact.
Generative AI offers great promise for crop breeding and genetic improvement. By analyzing large genomic datasets, AI models can identify genetic markers associated with desirable traits like disease resistance, yield potential, nutritional value etc. This allows breeders to make more informed decisions when selecting parent plants for crossbreeding and speed up the development of improved varieties of crop plants.
Although generative AI brings numerous advantages, challenges remain. Chief among them are data availability and quality; training accurate AI models requires large datasets with diverse information that may be difficult to come by in developing regions with limited connectivity or data infrastructure. There may also be issues regarding data privacy/ownership as well as ethical ramifications of using AI farming practices.
Request Sample Copy of Generative AI in Agriculture Market Report at: https://marketresearch.biz/report/generative-ai-in-agriculture-market/request-sample
Key Takeaways
- Generative AI for agriculture provides farmers with data-driven insights and recommendations for optimized crop management.
- Livestock management can gain tremendously from AI thanks to sensor data analysis that enables early disease detection and improved animal welfare.
- Precision agriculture can be further advanced through artificial intelligence (AI) models that integrate data from various sources to create detailed field maps and ensure efficient input distribution.
- Generative AI's capabilities of analyzing genomic datasets and recognizing desirable traits has hastened crop breeding and genetic improvement efforts.
- Data availability and quality present a major obstacle to adopting generative AI in agriculture, particularly in regions with limited connectivity and infrastructure.
- Concerns are being expressed over data privacy, ownership and ethical implications posed by using generative AI for farming practices.
- Generative AI holds great promise to enhance efficiency, sustainability and productivity in agriculture industries.
- Continued development and adoption of generative AI in agriculture holds great promise to address key challenges while driving innovation within the sector.
Regional Snapshot
North America is at the forefront of adopting artificial intelligence (AI) solutions in agriculture, thanks to advanced infrastructure, strong digital connectivity and a vibrant tech ecosystem. Many companies and research institutions are currently actively creating AI solutions for crop management, precision agriculture and livestock monitoring purposes in North America.
Europe is also making considerable strides forward with regards to applying generative AI to agriculture. Thanks to European Union initiatives promoting sustainable farming practices and digitalizing agriculture, more AI technologies are being adopted into farming operations than ever before – specifically those used for optimizing resource allocation, minimizing environmental impact and improving overall farm productivity. Generative AI helps optimize resource allocation while simultaneously reducing environmental impact while simultaneously increasing overall farm productivity.
Asia-Pacific region AI adoption is rapidly rising as countries like China, India and Australia turn towards AI for agricultural technology investments and use. Generative AI allows farmers to make data-driven decisions to maximize yields.
Latin American countries are starting to recognize the power of generative AI in agriculture. Boasting vast agricultural landscapes with diverse crops, Latin American countries could gain immensely from AI-powered insights. Generative AI research is being undertaken with regards to optimizing irrigation practices, monitoring soil health, and pest management – contributing towards more sustainable farming practices and increased productivity.
Africa has seen the adoption of generative AI for agriculture steadily increasing over time; though at a slower rate compared to other regions. Challenges related to connectivity and data infrastructure are being addressed through initiatives and partnerships aimed at closing digital divides. Generative AI holds great promise for smallholder farmers in Africa who depend on informed decisions for making informed decisions and increasing crop yields.
For any inquiries, Speak to our expert at: https://marketresearch.biz/report/generative-ai-in-agriculture-market/#inquiry
Drivers
Increasing demand for sustainable and efficient farming practices
As the global population is projected to reach 9 billion by 2050, there is an ever-increasing need for sustainable and efficient farming practices that meet rising food demands. Generative AI offers solutions designed to optimize resource allocation, reduce wastefulness and lessen the environmental impacts of agricultural activities – in line with the goals of sustainable agriculture.
Increased availability of agricultural data
Technological advances have given agriculture access to new data collection tools such as remote sensing, drones and IoT sensors for collecting information about soil moisture levels, temperature conditions, crop health status and weather conditions. Generative AI algorithms can then use this information to uncover patterns or detect anomalies to provide actionable insights for improved agriculture practices and allow data-driven decision-making by farmers.
Advancements in machine learning and computing power
Recently, advances in machine learning algorithms, such as deep learning and neural networks, have significantly enhanced the capabilities of generative AI models for agriculture. These models can process large agricultural datasets more accurately and rapidly for more precise predictions and recommendations. Furthermore, increased computing power and cloud infrastructure has facilitated the training and deployment of complex generative AI models in agriculture.
Government support and initiatives
Governments around the world have realized the potential of AI for agriculture and are actively supporting its adoption through various support mechanisms. These include funding research and development initiatives, creating regulatory frameworks, and initiating programs to educate farmers about AI's benefits in farming. Government involvement plays a pivotal role in driving the adoption and integration of generative AI technologies within agricultural environments.
Restraints
Limited Data Availability
One of the main stumbling blocks to using AI in agriculture is limited data availability. AI models rely on large datasets in order to produce accurate and reliable outputs; however, due to various constraints – limited access, privacy concerns, quality issues etc – acquiring such datasets in agriculture can be challenging due to limited representation and training/performance limitations of models created using generative AI.
Cost and Infrastructure Requirements
Implementing AI solutions into agriculture often requires substantial investments in terms of infrastructure, computational resources and data storage capabilities. Unfortunately, the high costs associated with setting up and maintaining this hardware/software infrastructure can be prohibitively costly for small-scale or resource-constrained agriculture operations; plus with constant updates/upgrades required to stay ahead of emerging AI technologies comes additional burdensome cost burden.
Ethical and Regulatory Challenges
Generative AI poses ethical and regulatory challenges to agriculture that must be met head on. Data generated by AI models poses complex ownership and privacy rights concerns for farmers and stakeholders who may worry about sharing it or misuse. Additionally, regulatory hurdles related to data protection, intellectual property rights, compliance with existing agricultural policies and regulations may impede widespread adoption of such technologies.
Interpretability and Explicability
Generative AI models such as deep learning-based neural networks may appear like black boxes due to their lack of transparency and interpretability, creating problems in agriculture where decisions based on AI-generated outputs must be fully justified by farmers and other stakeholders, creating trust barriers which inhibit adoption of these solutions and limit acceptance and adoption rates of such solutions.
Opportunities
Yield optimization and resource efficiency
Generative AI models can leverage various datasets, such as historical yield data, weather patterns and soil conditions to provide farmers with insights on optimal planting times, nutrient application rates and irrigation schedules that allow them to maximize crop yields while efficiently using resources like water, fertilizers and pesticides.
Disease and Pest Management Solutions
Early identification and timely intervention are integral components of effective disease and pest management in agriculture. Generative AI can analyze sensor data collected from farm equipment, drones and remote sensing technologies to detect patterns or anomalies associated with outbreaks or infestations and provide early warning to farmers who can then take preventive steps or apply targeted treatments accordingly, thus minimizing crop losses while simultaneously decreasing chemical input costs.
Crop breeding and genetic enhancement
Generative AI can quickly analyze genomic datasets to identify genetic markers associated with desirable traits in crops, such as disease resistance, drought tolerance or increased nutritional value. By understanding their genetic makeup, breeders can make informed decisions during crossbreeding programs to speed up the development of improved varieties that address specific challenges while meeting market demands.
Automation and robotics in agriculture
Integrating robotics and automation technologies with generative AI opens up opportunities for increased efficiency and lower labor requirements in agriculture. Generative AI powers autonomous systems, drones, and robots that perform tasks such as planting, harvesting, crop monitoring and weed management – improving operational efficiencies while decreasing labor costs while providing targeted actions within agricultural operations. These technologies enhance operational efficiencies as they ensure precise actions at harvesting time for farmers.
Take a look at the PDF sample of this report: https://marketresearch.biz/report/generative-ai-in-agriculture-market/request-sample
Challenges
Complex Agricultural Systems
Agriculture is an immensely complex field with multiple interdependent elements affecting crop growth, disease management, yield prediction, and other key aspects. Building accurate and robust generative AI solutions presents a considerable challenge given that so much must be considered when simulating agricultural systems – including soil conditions, weather patterns, pest and disease dynamics dynamics, genetics of crops etc.
Lack of Domain-Specific Expertise
Building effective generative AI solutions for agriculture requires an in-depth knowledge of both AI techniques and domain-specific agricultural knowledge, yet there is a scarcity of experts with this expertise in both fields. Bridging this knowledge gap and encouraging collaboration between AI researchers and agricultural domain experts can be difficult; without domain expertise it becomes harder to create models that truly address the unique challenges and requirements of the agriculture industry.
Scalability and Adaptability
Agriculture operations range in scale from small family farms to large commercial enterprises, making developing AI solutions that scale and adapt to these different farm sizes, crops and geographical regions a complex endeavor. Customizing AI models to fit specific farming contexts or requirements may take considerable time and resource investment; in addition, keeping up-to-date and fine-tuning them with changing environmental conditions or emerging agricultural practices poses another scalability hurdle.
Market Segmentation
Based on Crop Type
- Wheat
- Rice
- Corn
- Vegetables
- Other Crop Types
Based on Application
- Precision Farming
- Livestock Management
- Crop Management
- Soil Analysis
- Other Applications
Based on Technology
- Deep Learning
- Computer Vision
- Machine Learning
- Natural Language Processing
- Robotics
Based on End-User Industry
- Farmers
- Agriculture Technology Companies
- Agriculture Consultants
- Government Agencies
- Research Institutions
Key Players
- IBM Corp.
- Microsoft Corp.
- John Deere
- The Climate Corporation (a subsidiary of Bayer)
- Ag Leader Technology
- Trimble Inc.
- Prospera Technologies
- Descartes Labs
- Taranis
- Granular (a Corteva Agriscience company)
- Other Key Players
Report Scope
Report Attribute | Details |
Market size value in 2022 | USD 125 Mn |
Revenue Forecast by 2032 | USD 1083.9 Mn |
Growth Rate | CAGR Of 24.8% |
Regions Covered | North America, Europe, Asia Pacific, Latin America, and Middle East & Africa, and Rest of the World |
Historical Years | 2017-2022 |
Base Year | 2022 |
Estimated Year | 2023 |
Short-Term Projection Year | 2028 |
Long-Term Projected Year | 2032 |
Request Customization Of The Report: https://marketresearch.biz/report/generative-ai-in-agriculture-market/#request-for-customization
Recent Developments
- In 2022, Blue River Technology, a subsidiary of John Deere, unveiled their See & Spray Select precision sprayer. Utilizing computer vision and AI algorithms, this advanced agricultural technology uses real-time targeting of individual weeds with herbicide applications reducing herbicide usage while simultaneously decreasing environmental impacts while simultaneously increasing crop yield.
- In 2021, CropX, an Israeli agtech company, announced their acquisition of Dacom Farm Intelligence, a Dutch data-driven solutions company for agriculture. Through their merger, these two entities combine expertise in soil sensing technology, AI, machine learning, irrigation recommendations and advanced soil management techniques to offer farmers advanced soil management strategies and maximize crop productivity while optimizing water usage and optimizing crop productivity.
- In 2021, FarmWise Robotics of California recently received $14.5 Million in funding to continue development of its autonomous weeding robots. Utilizing AI and computer vision technology, these robotic weeders detect and remove weeds from fields with precision while eliminating chemical herbicides or manual labor from fields – significantly reducing manual labor requirements and herbicide costs. With this funding boost FarmWise can expand their fleet, furthering their mission for efficient sustainable agriculture management.
FAQ
1. What are the characteristics of generative AI for agriculture?
A. Generative AI in agriculture refers to the application of artificial intelligence techniques utilizing generative models. Such models can generate new data samples or simulate complex agricultural systems for tasks like yield prediction, disease detection and resource allocation optimization.
2. How can generative AI technology benefit the agriculture industry?
A. Generative AI offers many advantages to the agriculture industry. It can assist with improving crop yield predictions, optimizing resource utilization, detecting and preventing diseases, simulating agricultural scenarios and providing data-driven insights for decision-making. Generative AI also has the potential to boost productivity while simultaneously cutting costs while supporting more eco-friendly practices in agriculture.
3. What are some examples of artificial intelligence applications in agriculture?
A. Generative AI applications in agriculture span an array of tasks, such as producing synthetic images for training computer vision models, simulating weather patterns that impact crop growth, providing recommendations for optimal irrigation and fertilizer application practices, as well as creating virtual environments to test and optimize robotic farming systems.
4. What are the challenges associated with implementing generative AI into agriculture?
A. Implementing generative AI in agriculture presents many obstacles, such as limited data availability, high infrastructure costs, ethical and regulatory concerns and the requirement of interpretable AI outputs. Furthermore, agricultural systems' complexity, lack of domain-specific expertise as well as variable quality data all present significant obstacles.
5. How can generative AI models be trained for agriculture applications?
A. Generative AI models in agriculture can be trained using various approaches. One popular strategy involves gathering large and varied datasets that contain information on crops, weather patterns, soil conditions, and other relevant factors. From there, deep learning techniques like GANs or VAEs may be employed to recognize patterns within input data or even generate samples based on input data.
6. Can Generative AI support sustainable agriculture practices?
A. Absolutely, generative AI can make a positive contribution to sustainable agriculture practices. By analyzing and simulating agricultural systems, generative AI can optimize resource allocation, reduce wastefulness, improve crop management practices, and aid in the creation of precision agriculture techniques that enable farmers to make informed decisions while mitigating environmental impact.
7. What are the prospects for generative AI applications in agriculture?
A. Future prospects of generative AI in agriculture look promising. As technology progresses and more agricultural data becomes available, generative AI models should become increasingly accurate and robust – leading to increased adoption in various agricultural domains resulting in improved productivity, resource efficiency and sustainability within the industry.
Contact us
Contact Person:ย Mr. Lawrence John
Marketresearch.Biz
Tel:ย +1 (347) 796-4335
Send Email:ย [email protected]
Content has been published via 11press. for more details please contact at [email protected]

The team behind market.us, marketresearch.biz, market.biz and more. Our purpose is to keep our customers ahead of the game with regard to the markets. They may fluctuate up or down, but we will help you to stay ahead of the curve in these market fluctuations. Our consistent growth and ability to deliver in-depth analyses and market insight has engaged genuine market players. They have faith in us to offer the data and information they require to make balanced and decisive marketing decisions.