Generative AI in Market Research: Unlocking Insights and Streamlining Decision-making
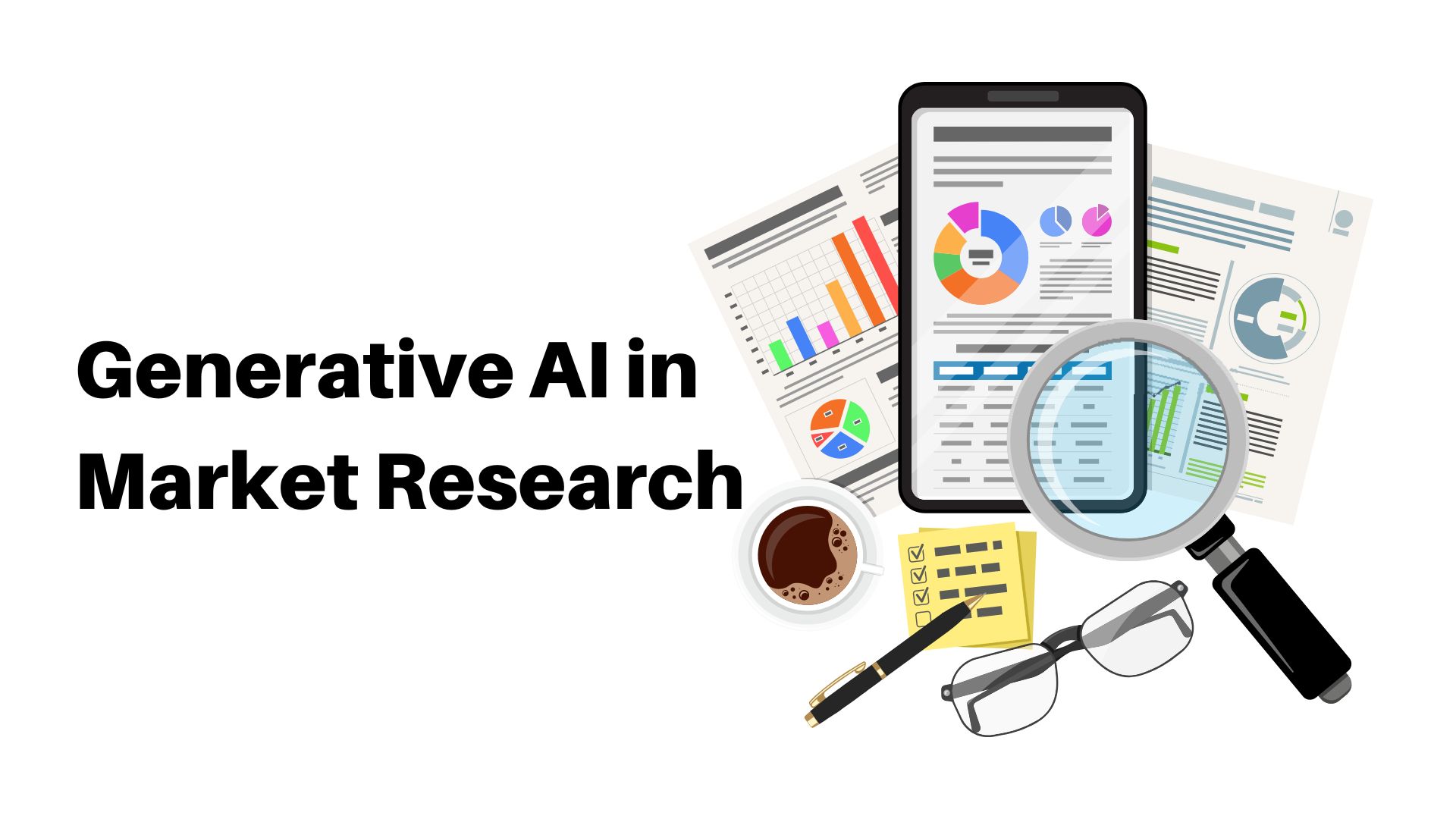
Page Contents
- Introduction:
- Generative AI in Market Research:
- Benefits of Generative AI:
- Applications of Generative AI in Market Research:
- Challenges of Generative AI in Market Research:
- Pros of Generative AI in Market Research:
- Cons of Generative AI in Market Research:
- Risks of Generative AI in Market Research:
- Conclusion:
Introduction:
In the realm of artificial intelligence (AI), generative AI has become the center of attention, revolutionizing various industries and reshaping the way we live and work. Market research is no exception, as generative AI presents an opportunity to delve deeper into understanding the needs and desires of target audiences. By rapidly and cost-effectively generating high-quality data, generative AI offers a powerful tool to unearth insights that might otherwise go unnoticed. This article explores the potential applications of generative AI in market research, with a focus on its ability to create predictive models, detect patterns, and provide real-time insights into consumer trends. By harnessing the capabilities of generative AI, market researchers can gain a profound understanding of their target audiences and make informed decisions regarding their offerings.
Generative AI in Market Research:
Generative AI refers to AI systems capable of self-generation, creating new information and ideas without explicit programming. Companies like YouGov have developed software solutions that leverage machine learning and natural language processing to enable researchers to generate new data without conducting additional empirical research. Market researchers can utilize generative AI to generate fresh data, leading to valuable insights about consumers, trends, and behaviors. For instance, generative AI can be employed to produce data on consumers' attitudes towards a product or service, which can then be used to develop predictive models and gain insights into purchasing trends.
Benefits of Generative AI:
- Increased Accuracy:
Generative AI outperforms other data collection methods, such as expensive social listening platforms, by producing more realistic views of target audiences. This enhanced accuracy allows researchers to gain deeper insights into consumer attitudes and behaviors. - Rapid Results:
Generative AI accelerates the process of generating new data, enabling researchers to initiate insights at a faster pace. This speed is facilitated by artificial neural networks, which efficiently process vast amounts of data and complex algorithms. - Cost-effectiveness:
Generative AI eliminates the need for additional empirical research, saving time and resources for organizations. As a result, research budgets can be optimized, promoting cost-effectiveness. - Convenience:
Generative AI offers a user-friendly experience, enabling anyone with access to the software, such as ChatGPT, to generate new data effortlessly. The software's algorithms are designed to be user-friendly, ensuring convenience in generating data.
Applications of Generative AI in Market Research:
- Predictive Modeling:
Predictive modeling utilizes historical data to forecast future outcomes. Market researchers can employ predictive modeling to gain insights into various areas, including buying and selling decisions, competition analysis, and product performance evaluation. - Pattern Detection:
Pattern detection uncovers hidden connections and correlations within different variables. By exploring consumer attitudes and behaviors, researchers can discover meaningful patterns that would remain hidden otherwise. Pattern detection can be applied to topics such as consumer behavior, preferences, and product performance. - Real-time Insights:
Real-time insights are derived from live data and are particularly useful for dynamic metrics such as social media and website traffic. Researchers can leverage real-time insights to gain a deeper understanding of consumer behavior, product performance, and competitive landscapes.
Challenges of Generative AI in Market Research:
- Scalability:
Generating new data can be a time-consuming process, potentially resulting in data not being readily available when needed. This can impact the accuracy of research and lead to delays. - Accuracy:
Generated data may not be as accurate as data collected through empirical research. Researchers must exercise caution when using generative AI data to gain insights into their target audiences. - Interpretability:
The process by which data is transformed from raw to usable form lacks transparency in generative AI. This limits researchers' understanding of how the data was generated, reducing its overall usefulness. - Dependability:
Using generated data may not be as reliable as relying on data collected through empirical research. Researchers need to carefully interpret and apply the data, and be prepared to question its accuracy.
Pros of Generative AI in Market Research:
- Increased accuracy: Generative AI can generate data more accurately compared to other data collection methods. It provides a more realistic view of target audiences, enabling researchers to gain a deeper understanding of consumers' attitudes and behaviors.
- Rapid results: Generative AI can quickly produce new data, allowing researchers to generate insights sooner. This speed is possible due to artificial neural networks that can process large amounts of data and complex algorithms.
- Cost-effectiveness: Generative AI allows researchers to create new data without the need for additional empirical research or expensive platforms. This saves time and money, allowing organizations to allocate their research budgets more efficiently.
- Convenience: Generative AI offers a user-friendly experience, making it accessible to anyone with access to the software. The algorithms used in the software are designed to be extremely user-friendly, enabling easy generation of new data.
Cons of Generative AI in Market Research:
- Scalability: Generating new data can be a time-consuming process, which may result in data not being readily available when needed. This could lead to lower accuracy and research delays.
- Accuracy: Generated data may not be as accurate as data collected through empirical research. Researchers must exercise caution when using generated data to understand target audiences.
- Interpretability: The process by which data is converted from raw to usable form with generative AI is not entirely transparent. Users have limited insight into how the data was generated, which can limit its usefulness.
- Dependability: Using generated data may not be as reliable as using data collected through empirical research. Researchers must be cautious when interpreting and applying the data, and be prepared to question its accuracy.
Risks of Generative AI in Market Research:
- Prompt dependency: Generative AI relies on prompts to generate responses. If the prompts are incorrect or misleading, the generated data may not provide accurate insights. Users need to be mindful of the input they provide to ensure reliable results.
- Trustworthiness: Generative AI has the potential to blend correct and incorrect information, which can lead to misleading or inaccurate results. It is essential to critically evaluate the generated data and verify its validity before making important business decisions.
- Lack of transparency: The algorithms used in generative AI may not always provide transparency regarding how the data was generated. Users may not have a clear understanding of the sources and processes used, limiting their ability to assess the reliability and validity of the generated data.
- Security concerns: Inputting sensitive company information into generative AI tools can pose security risks. There is a possibility of exposing confidential data or intellectual property to unauthorized access. Organizations need to ensure strict data security measures and adhere to internal data protection policies when using generative AI.
- Bias and fairness: Generative AI systems can inherit biases from the data they are trained on, which can result in biased outputs. It is crucial to be aware of potential biases in the generated data and take steps to mitigate them to ensure fair and unbiased insights.
- Interpretation challenges: The process of converting raw data into usable form with generative AI may not always be transparent. This can make it difficult to understand how the data was generated and limit researchers' ability to interpret and analyze the data effectively.
It's important for organizations to be aware of these risks and take necessary precautions when leveraging generative AI in market research. This includes rigorous validation of generated data, transparency in data generation processes, and adherence to data privacy and security protocols.
Conclusion:
While some fear that AI may replace human involvement in market research, a more optimistic perspective recognizes the potential for AI to create new opportunities and jobs. By adopting and harnessing generative AI, researchers can gain a better understanding of their target audiences, leading to more informed decisions regarding their offerings. Rather than fearing AI's potential disruption, it is crucial to embrace its possibilities and utilize it to our advantage. As the famous quote goes, “With great power comes great responsibility.” It is up to us to leverage generative AI responsibly and use it as a tool for progress in market research.

Barry is a lover of everything technology. Figuring out how the software works and creating content to shed more light on the value it offers users is his favorite pastime. When not evaluating apps or programs, he's busy trying out new healthy recipes, doing yoga, meditating, or taking nature walks with his little one.