Generative AI in Science Market to Hit USD 45.9 Bn by 2032
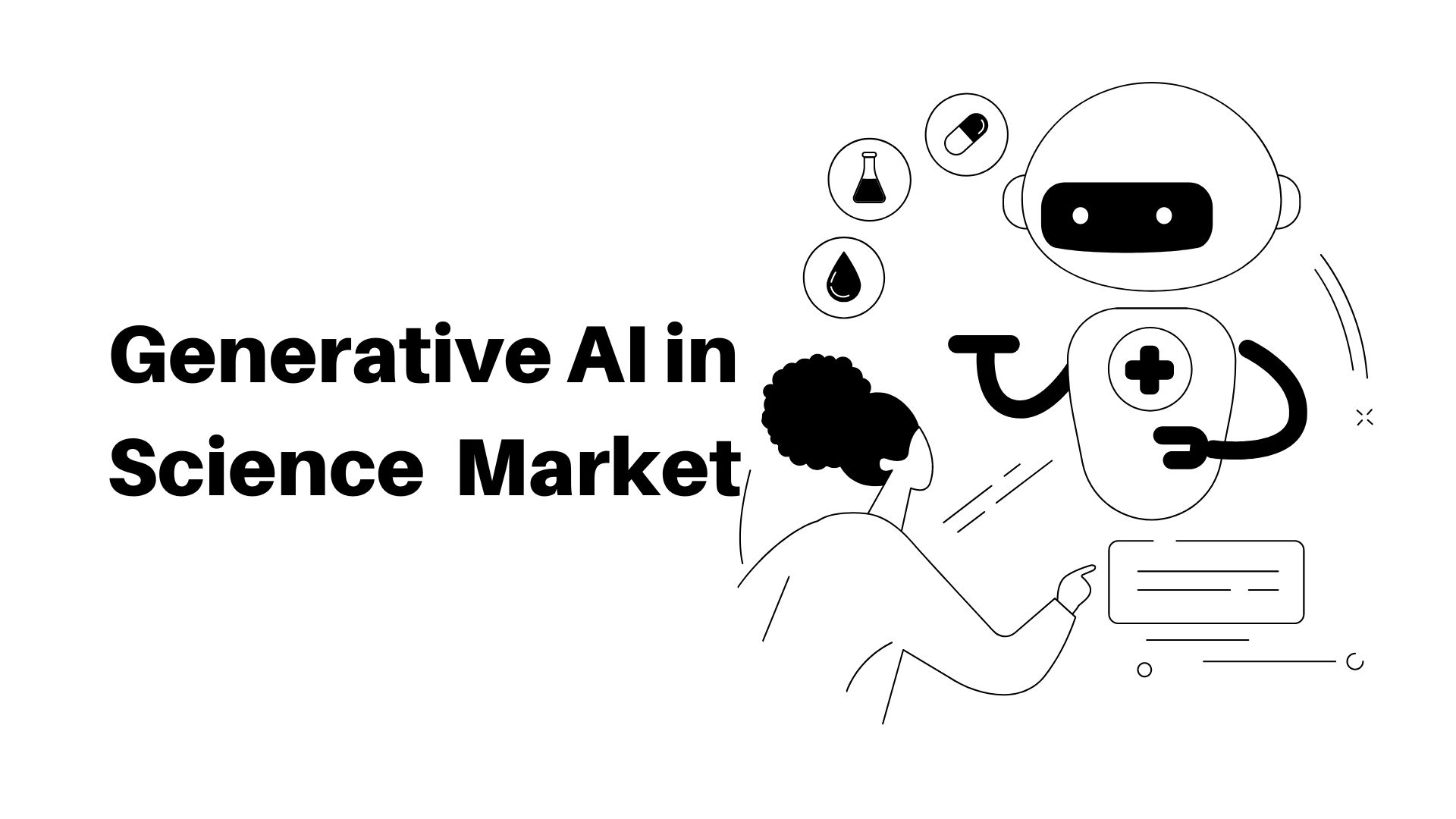
Page Contents
Market Overview
Published Via 11Press : Generative AI in Science Market size is expected to be worth around USD 45.9 Bn by 2032 from USD 3.2 Bn in 2022, growing at a CAGR of 31.4% during the forecast period from 2022 to 2032.
Generative artificial intelligence (AI) in science has experienced rapid expansion. Generative AI refers to using machine learning algorithms to generate content like images, texts or scientific hypotheses from existing data patterns and examples – revolutionizing research while speeding innovation across many fields. Generative AI technology has revolutionized research while speeding innovation at unprecedented rates.
Generative AI has proven itself invaluable in drug discovery and development. Pharmaceutical companies are using AI algorithms to quickly design new medicines with desirable properties by analyzing databases of chemical compounds with known biological activities – an approach which has the potential to speed up discovery processes, lower costs and facilitate innovation of treatments more rapidly than ever before.
Materials science is another area where generative AI is making waves, with researchers using predictive and design models to create new materials with specific properties such as increased strength or conductivity. Generative AI algorithms have the capacity to quickly scan through vast collections of potential material compositions and structures in order to quickly identify promising candidates for further experimentation; this technology could revolutionize development of advanced materials used for electronics, energy storage or catalysis applications.
Generative AI has also made waves in computational biology and genomics research. Researchers can utilize generative models to simulate biological systems like protein folding or gene expression and gain insights into complex biological processes by simulating their interactions – in turn creating virtual models for further investigation. With this approach comes potential advancement of our knowledge about fundamental biological mechanisms while aiding personalized medicine development simultaneously.
Generative AI has also proven invaluable when it comes to data analysis and interpretation. Scientists use generative models to generate synthetic data that mimics real-world observations in order to test analytical methods. This allows researchers to gain a better understanding of complex datasets, identify patterns more easily, and extract meaningful insights more quickly than before. Furthermore, AI algorithms such as this one can assist when collecting large amounts of labeled data is difficult or time-consuming.
Request Sample Copy of Generative AI in Science Market Report at: https://marketresearch.biz/report/generative-ai-in-science-market/request-sample
Key Takeaways
- Generative AI science is revolutionizing drug discovery by creating novel molecules with desirable therapeutic properties.
- Generative AI is revolutionizing materials science by speeding up the creation of advanced materials for various uses.
- Computational biology and genomics have made great advances thanks to artificial intelligence, making their understanding of complex biological processes much simpler.
- Generative AI provides scientists with assistance for data analysis and interpretation, helping them uncover meaningful insights from large datasets.
- Recent advances in AI algorithms and computing power are driving an explosion in generative AI research.
- Collaboration among academic institutions, research organizations, and technology companies is driving advances in generative AI.
- Generative AI could transform scientific research processes by cutting costs and shortening time required for discovery.
- Due to its wide-ranging applications and advantages, generative AI in science is forecasted to experience rapid expansion over the coming years.
Regional Snapshot
North America remains an epicenter for technological innovation, particularly within the US. Silicon Valley continues to drive advances in artificial intelligence, biotechnology, and information technology; additionally, renewable energy research and sustainability initiatives remain prominent areas.
Europe boasts an outstanding scientific and research community. Countries like Germany, Britain and France are known for their contributions in fields like automotive engineering, aerospace technology and pharmaceutical research. Furthermore, the European Union fosters collaboration and funding of cutting-edge initiatives related to climate change mitigation and digital transformation research projects.
Asia-Pacific region is witnessing dramatic advancement in science and technology, particularly China which stands out as a leader in research and development with particular attention paid to emerging technologies like artificial intelligence, quantum computing and genomics. Japan excels in robotics manufacturing while countries such as South Korea and Singapore prioritize digitalization of society and innovation.
Latin America is experiencing an upsurge in interest for science and technology. Brazil leads with investments in biofuels, agricultural research and space exploration while Mexico and Chile have made strides in renewable energy research as well as environmental sciences. Furthermore, efforts are underway to enhance science education as well as drive entrepreneurialism across Latin America – stimulating innovation throughout this region.
Africa is becoming an international center for scientific advancement. South Africa stands out as an innovator, hosting world-class research institutions and making notable strides in areas like astronomy, biodiversity and renewable energy research. Kenya, Nigeria and Senegal are also investing in science and technology as a means of driving economic growth while meeting regional challenges.
For any inquiries, Speak to our expert at: https://marketresearch.biz/report/generative-ai-in-science-market/#inquiry
Drivers
Data Augmentation and Creation
Generative AI models offer researchers a powerful tool for augmenting and creating data in the scientific domain. By training on existing datasets, these models can produce synthetic information that closely resembles real data – helping researchers overcome data limitations, increase sample sizes and investigate broader scenarios, leading to greater scientific insight and understanding.
Novel Experiment Design
Generative AI models can help optimize experiment design by simulating virtual experiments. These models can explore an expansive parameter space and generate various experimental scenarios, helping researchers identify optimal conditions, reduce costs, and accelerate discovery in various scientific fields.
Hypothesis Generation and Exploration
Generative AI models can assist scientists in hypothesis generation and exploration by offering plausible alternative hypotheses or scenarios. By drawing upon learned patterns from existing data sources, these models can also propose novel hypotheses which researchers can further investigate, potentially leading to breakthrough discoveries and breakthroughs in scientific research.
Drug Discovery and Material Design.
Generative AI holds great promise in drug discovery and material design. By creating molecular structures, these models can assist with exploring chemical space to identify promising drug candidates or novel materials with desired properties – speedsing the development process and revolutionizing pharmaceutical and materials science industries alike.
Restraints
Provide Access and Quality Data Solutions
One of the main stumbling blocks to using generative AI in scientific domains is availability and quality of data. Generative models often require large volumes of high-quality training data in order to produce accurate and reliable results; however, due to limited availability, privacy concerns or costly data collection processes this may prove challenging in science domains. Poor-quality or insufficient amounts can have serious repercussions for performance and reliability of scientific applications using generative AI models.
Ethical Considerations
Generative AI models have the capacity to generate synthetic data that closely resembles real world information, which may prove advantageous in certain instances; however, this raises ethical concerns that could potentially compromise scientific research through misrepresentation or misuse of this generative AI-generated data and lead to false or erroneous conclusions or unethical practices. Ensuring responsible use and compliance with ethical guidelines when producing or using synthetic data remains a significant challenge in science markets today.
Interpretability and Explainability
Generative AI models such as deep neural networks often operate like black boxes, making it challenging to interpret and explain their decision-making processes. Interpretability and explainability are vital tools in scientific applications as it helps researchers validate generated results, so generative models that lack transparency may pose significant restrictions especially where accuracy of generated data or insights is paramount.
Generalizing and Transfer Learning
Generative AI models trained on specific scientific datasets may struggle to apply their knowledge across to new situations that arise unexpectedly, limiting its utility and applicability within the science market. Addressing this challenge by enabling transfer learning across scientific domains remains an ongoing research area that must be explored for wide adoption of generative AI models.
Opportunities
Personalized Medicine and Treatment Options Available Now
Generative AI models can be leveraged to develop personalized medicine and treatment strategies. By analyzing patient data such as genetic information, medical records, lifestyle factors and prognosis information derived from patient surveys or genetic testing results generative AI can generate personalized models and predictions to assist in disease diagnosis, treatment selection and outcome prognosis – revolutionizing healthcare while improving patient outcomes.
Simulation and Predictive Modeling
Generative AI can be leveraged to generate realistic simulations and predictive models in multiple scientific disciplines. By learning from existing data, generative models can produce synthetic data to simulate complex phenomena such as climate patterns, fluid dynamics, or protein folding – providing invaluable insight and supporting decision-making processes.
Data Imputation and Reconstruction (DIAR)
Scientific research can be made more complex by missing or incomplete data points that impede analysis and interpretation. Generative AI models provide researchers with a way to fill these missing gaps in order to improve dataset completeness and quality; this enables more accurate inferences, more rigorous statistical analysis and meaningful conclusions from incomplete datasets.
Image and Signal Processing Services
Generative AI techniques excel in image and signal processing tasks. Used across scientific fields like astronomy, microscopy and remote sensing generative models can enhance image quality while simultaneously denoising signals and reconstructing high-resolution images from low-resolution inputs – dramatically improving data analysis and visualization and helping scientists extract vital information from complex imaging/sensing datasets.
Take a look at the PDF sample of this report: https://marketresearch.biz/report/generative-ai-in-science-market/request-sample
Challenges
Knowledge of the Scientific Domain
Generative AI models designed for scientific applications require expertise both in AI techniques and the respective scientific field. Understanding its underlying principles, experimental procedures, and domain-specific constraints is paramount in creating effective generative models. Bridging the divide between AI experts and domain scientists and encouraging cross-disciplinary collaboration are formidable obstacles.
Algorithmic Robustness
Generative AI models can be highly susceptible to variations in input data, leading to unpredictable and inconsistent outputs. Ensuring algorithmic robustness and stability is paramount in scientific applications where accuracy and reproducibility are essential; creating techniques to address the robustness challenge and increase reliability is an ongoing area of research.
Scalability and efficiencyย
Scaling generative AI models to handle large and complex scientific datasets while maintaining computational efficiency is a daunting challenge. As scientific data volumes and complexity increase, developing scalable generative AI approaches becomes even more essential. Effective training/inference algorithms, model architectures, and distributed computing techniques are being explored as possible solutions to tackle scaling difficulties.
Integration into Existing Workflows
Integration of generative AI models seamlessly into existing scientific workflows and tools can be challenging since scientists often rely on established software, methodologies, and data formats when it comes to adopting these techniques. Ensuring smooth integration and encouraging adoption is a challenge that must be tackled before success can be reached with this field of AI research.
Market Segmentation
Based on the Deployment Model
- Cloud-based
- On-Premises
Based on Application
- Drug Discovery
- Material Science
- Medical Imaging and Healthcare
- Astrophysics and Astronomy
- Molecular Biology
- Other Applications
Based on End-User
- Pharmaceutical and Biotechnology companies
- Research Institutions and Academic Institutions
- Healthcare Providers
- Government Organizations
- Other End-Users
Key Players
- NVIDIA
- Insilico Medicine
- Atomwise
- Recursion Pharmaceuticals
- Intel
- Yseop
- BenevolentAI
- Other Key Players
Report Scope
Report Attribute | Details |
Market size value in 2022 | USD 3.2 Bn |
Revenue Forecast by 2032 | USD 45.9 Bn |
Growth Rate | CAGR Of 31.4% |
Regions Covered | North America, Europe, Asia Pacific, Latin America, and Middle East & Africa, and Rest of the World |
Historical Years | 2017-2022 |
Base Year | 2022 |
Estimated Year | 2023 |
Short-Term Projection Year | 2028 |
Long-Term Projected Year | 2032 |
Request Customization Of The Report: https://marketresearch.biz/report/generative-ai-in-science-market/#request-for-customization
Recent Developments
- In 2023, Google AI recently unveiled Pathways, an ambitious research initiative dedicated to finding new methods of creating synthetic data for training machine learning models.
- In 2023, Anthropic announceds its development of Universe, an artificial intelligence (AI)-powered research platform designed for scientific study.
- In 2023, Stability AI announced the creation of MedGAN, an AI-powered generative platform designed specifically for medical imaging.
FAQ
1. What Is Generative AI in Science Market?
A. Generative AI refers to artificial intelligence techniques which generate new content similar to real world examples. Generative AI techniques are frequently utilized by scientists for data generation, hypothesis testing, drug discovery and personalized medicine as well as simulations.
2. What are the advantages of Generative AI in scientific research?
A. Generative AI assists with producing synthetic data, discovering novel insights, simulating experiments, optimizing designs, speeding drug discovery, expediting personalized medicine care delivery, compressing data storage requirements and improving image and signal processing.
3. What challenges does Generative AI encounter in the science market?
A. Challenges associated with data availability include ethical considerations, interpretability, generalization, computational resources integration domain-specific knowledge algorithmic robustness scalability and validation.
4. How does Generative AI contribute to drug discovery and materials design?
A. Generative AI creates novel molecular structures, aiding exploration of chemical space, identifying potential drug candidates, optimizing molecular properties and speeding drug discovery and materials design processes.
5. Can Generative AI complement personalized medicine?
A. Generative AI analyzes patient data to generate personalized models and predictions, aiding with disease diagnosis, treatment selection, outcome prediction and personalized healthcare recommendations.
6. How does Generative AI enhance data analysis and visualization?
A. Generational AI techniques enhance image quality, denoise signals, reconstruct high-resolution images and enable improved data analysis and visualization for scientific research, such as in astronomy or microscopy.
7. Can Generative AI facilitate collaborative research and knowledge sharing?
A. Yes, Generative AI allows collaborative research by producing synthetic datasets while protecting data privacy. Furthermore, this technology also facilitates synthetic research papers, encouraging interdisciplinary cooperation and knowledge exchange among scientists.
Contact us
Contact Person:ย Mr. Lawrence John
Marketresearch.Biz
Tel:ย +1 (347) 796-4335
Send Email:ย [email protected]
Content has been published via 11press. for more details please contact at [email protected]

The team behind market.us, marketresearch.biz, market.biz and more. Our purpose is to keep our customers ahead of the game with regard to the markets. They may fluctuate up or down, but we will help you to stay ahead of the curve in these market fluctuations. Our consistent growth and ability to deliver in-depth analyses and market insight has engaged genuine market players. They have faith in us to offer the data and information they require to make balanced and decisive marketing decisions.