Generative AI in Testing Market to Witness Positive Growth at 17.2% CAGR
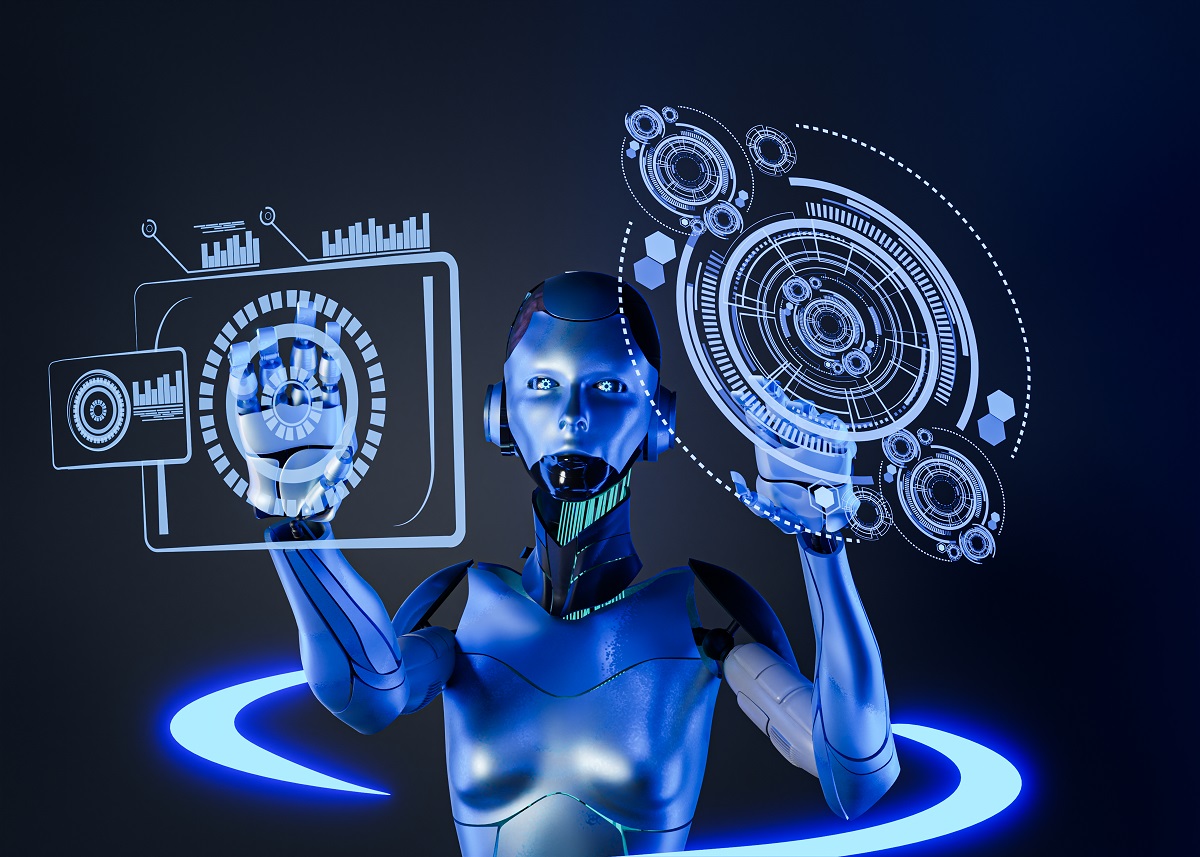
Page Contents
Market Overview
Published Via 11Press : Generative AI in Testing Market size is expected to be worth around USD 150.4 Mn by 2032 from USD 32 Mn in 2022, growing at a CAGR of 17.2% during the forecast period from 2022 to 2032.
Generative AI testing has seen rapid expansion and adoption. Also known as artificial intelligence that creates new content, generative AI has rapidly gained acceptance within software testing processes to automate and enhance testing activities. Utilizing machine learning algorithms for test data generation and script creation, this technology reduces manual effort while improving efficiency and saving time and effort in manual testing environments.
One of the key factors driving growth of the generative AI in testing market is the rising complexity of software systems. Thanks to cloud computing, big data, and IoT applications becoming ever-more intricate requiring stringent testing for reliability and functionality – an issue Generative AI is adept at solving through its ability to generate realistic test scenarios that cover various use cases and edge cases allowing comprehensive testing and improved software quality.
Another factor contributing to market expansion is the need for faster and more efficient testing processes. Manual methods may take too much time and labor for large-scale software projects; with Generative AI in testing accelerating testing processes by automating test case generation, freeing testers up for more important tasks like analysis and debugging while speeding time-to-market and cutting development costs significantly faster.
Generative AI provides many advantages in testing, such as increased test coverage and accuracy. By creating diverse test scenarios – even those rarely found elsewhere – this technology helps uncover defects or vulnerabilities that would go undetected with traditional methods. Furthermore, its adaptability enables more accurate predictions as well as easier identification of potential issues.
The generative AI testing market is experiencing rapid expansion, with numerous solution providers providing tools and platforms for software systems being tested. Many of these solutions integrate easily with existing testing frameworks and tools for seamless software development lifecycle integration; many also include AI algorithms that learn and adapt over time as requirements change for testing software systems being examined.
However, adopting generative AI for testing does present its own set of unique challenges and considerations. Ethical considerations pertaining to biased or discriminatory test data generation need to be carefully managed, while quality and reliability assurance for the models themselves is of utmost importance as any errors or biases could lead to inaccurate test results.
Request Sample Copy of Generative AI in Testing Market Report at: https://marketresearch.biz/report/generative-ai-in-testing-market/request-sample
Key Takeaways
- Generative AI testing is on the rise due to software's increasing complexity and the resulting need for thorough QA practices.
- Automation delivers faster and more efficient testing processes by streamlining test case and data generation processes.
- Generative AI increases test coverage and accuracy by creating realistic test scenarios.
- Market observers have witnessed a rapid proliferation of solution providers offering integrated tools and platforms.
- Ethics and quality assurance of generative AI models should both be given careful consideration prior to adoption.
- Generative AI testing improves software quality, reduces time-to-market and development costs while simultaneously increasing speed to market.
- Testing platforms enable testers to concentrate on core activities like analysis and debugging, thus increasing productivity.
- Continuous learning and adaption capabilities of generative AI models contribute to their efficiency and scalability in testing environments.
Regional Snapshot
North America is an established market for generative AI in testing, featuring major technology firms with an eye toward innovation. There has been significant adoption across industries including IT, healthcare, finance and automotive.
Europe has emerged as an ideal market for generative AI-powered testing due to the region's increased emphasis on digital transformation and software quality. Countries like Germany, UK and France have seen increased adoption of generative AI testing tools to both increase software reliability and speeding up testing processes.
Asia Pacific region is witnessing fast expansion in the generative AI testing market. Countries like China, India and Japan have invested significantly in technology and adopted generative AI for increasing software testing capabilities. A robust IT sector and increasing emphasis on quality assurance drive market growth in this region.
Latin America has seen increased adoption of generative AI testing technologies, particularly in Brazil and Mexico. Industries including telecom, banking, and e-commerce are turning to AI for testing purposes to streamline testing activities and boost software performance.
Middle East and Africa countries are gradually exploring the advantages of using generative AI for testing. Countries like UAE and South Africa are showing great interest in adopting this technology to both increase software quality and reduce testing times.
For any inquiries, Speak to our expert at: https://marketresearch.biz/report/generative-ai-in-testing-market/#inquiry
Drivers
Software Systems Are Becoming Ever Complex
As software systems become increasingly complex, their testing requirements become more extensive. Generative AI testing offers the ability to quickly generate diverse and realistic test scenarios covering numerous use cases and edge cases to increase the quality and reliability of software products.
Demand for Faster and Efficient Testing Processes
Generative AI testing methods offer an effective solution that's more time and labor efficient for large software projects, with traditional manual methods becoming time-consuming and labor-intensive over time. Generative AI can automate test case generation and data creation to accelerate testing efforts while freeing testers up for more strategic tasks like analysis or debugging, speeding time to market while decreasing development costs.
Improve Test Coverage and Accuracy
Generative AI testing provides a range of test scenarios, from rare and complex ones that might otherwise go undetected, that cannot be identified using traditional approaches to increased test coverage and accuracy for defect and vulnerability identification – ultimately improving overall software quality.
Integration with Existing Testing Frameworks and Tools
The market for generative AI in testing offers organizations a range of solutions that integrate seamlessly with existing testing frameworks and tools, allowing them to reap its advantages without disrupting current testing processes. Furthermore, many solutions come equipped with intelligent algorithms which continuously learn and adapt over time in response to changes within the software systems being tested.
Restraints
Ethical Considerations
Generative AI testing raises ethical concerns, such as discriminatory or biased test data generation. To effectively manage these worries and ensure the generated test data reflect real-world scenarios to prevent biases from impacting testing results, care must be taken in managing them to prevent potential imbalances that might alter the results of testing results.
Generative AI Model Quality and Reliability Analysis
Effective generative AI testing depends heavily on the quality and reliability of its AI models, with any errors or biases leading to flawed test results that compromise the reliability of testing processes. Organizations should invest in rigorous model validation processes as well as ongoing monitoring and improvement for all the AI models used for testing purposes.
Implementing Generative AI Requires Expertise
Utilizing Generative AI requires expertise in AI and machine learning technologies. Unfortunately, organizations may experience a shortage of skilled personnel capable of effectively using Generative AI for testing processes. Therefore, the investment must be made in training and development programs in order to bridge any knowledge or skills gaps and ensure the successful adoption of Generative AI testing processes.
Resistance to Change
Adopting generative AI testing methods may meet resistance from stakeholders who are used to traditional methods, as there may be hesitation about adopting new technologies – hindering its widespread adoption. Implementation of change management and communication strategies are therefore vital to overcome any resistance, while simultaneously highlighting its benefits.
Opportunities
Adoption in Different Industries
Generative AI testing offers potential adoption across industries such as IT, healthcare, finance and automotive. Each sector can realize cost-savings due to generative AI's automation and efficiency improvements during testing processes.
Emerging Markets Continue Their Strong Performance
Emerging markets such as Southeast Asia, South America and parts of Africa are experiencing exponential software development growth. This presents ample opportunity for adopting generative AI in testing to enhance software quality and testing efficiency for organizations within these regions.
Progresses in AI Technology
AI technology continues to advance at an incredible pace, particularly in generative models and machine learning algorithms, offering opportunities for greater capabilities of testing generative AI models. As AI evolves further there will be greater potential to create more sophisticated and accurate generative models for testing purposes.
Integrate into DevOps and Agile practices
Generative AI testing aligns closely with DevOps and Agile approaches that place an emphasis on continuous integration, testing, and delivery. There is an opportunity to incorporate generative AI into these workflows for seamless testing across the software development lifecycle.
Take a look at the PDF sample of this report: https://marketresearch.biz/report/generative-ai-in-testing-market/request-sample
Challenges
Data Privacy and Security
Generative AI testing requires access to large amounts of data for training its models, making data privacy and security an increasingly pressing challenge for organizations. Organizations should implement robust data protection measures as part of compliance efforts with relevant privacy regulations.
Explainability and Transparency
Generative AI models often operate like black boxes, making it difficult to understand how they generate test data and make decisions. Lack of explainability and transparency can act as an impediment to adoption in industries with strict accountability requirements; especially where accountability must be proven clearly through audit trails.
Cost and Infrastructure Requirements
Implementing generative AI testing may require significant investments in infrastructure, computational resources and AI talent. Acquiring and maintaining these resources may present some organizations, especially smaller enterprises with specific budget restrictions, with difficulties.
Continued Model Improvement and Adaptation
Generational AI models used in testing must constantly learn and adapt to changes in software systems. Ensuring their continuous improvement can be complex; dedicating dedicated resources for monitoring, updating, retraining, and maintaining these models to ensure optimal effectiveness in dynamic testing environments is necessary.
Market Segmentation
Based on Testing Types
- Functional Testing
- Performance Testing
- Security Testing
- User Interface Testing
- Other
Based on Industry
- IT and Telecommunications
- Finance
- Retail
- Automotive
- Healthcare
- Other
Based on the Deployment Mode
- On-premises
- Cloud-based
Based on Applications
- Test Case Generation
- Bug Detection and Diagnosis
- Test Data Generation
- Test Environment Simulation
- Intelligent Test Prioritization
Key Players
- Applitools
- Functionize
- Mabl
- Diffblue
- AdaLogica
- Appvance
- ai
- Other
Report Scope
Report Attribute | Details |
Market size value in 2022 | USD 32 Mn |
Revenue Forecast by 2032 | USD 150.4 Mn |
Growth Rate | CAGR Of 17.2% |
Regions Covered | North America, Europe, Asia Pacific, Latin America, and Middle East & Africa, and Rest of the World |
Historical Years | 2017-2022 |
Base Year | 2022 |
Estimated Year | 2023 |
Short-Term Projection Year | 2028 |
Long-Term Projected Year | 2032 |
Request Customization Of The Report: https://marketresearch.biz/report/generative-ai-in-testing-market/#request-for-customization
Recent Developments
- In 2021, Functionize, an independent testing company specializing in autonomous testing, introduced its generative testing capability. Utilizing machine learning algorithms, Functionize's generative testing technology enables organizations to achieve comprehensive coverage in their testing efforts through its intelligent test case generation technology.
- In 2022, Appsurify, a software testing company, unveiled their generative testing solution. Using AI algorithms to automatically create test cases and reduce manual labor hours; organizations can utilize this to increase coverage while shortening testing cycles and accelerating cycle times.
- In 2022, Testim An AI-powered test automation platform provider, Testim introduced its generative AI capabilities. Utilizing machine learning algorithms and artificial neural networks, their generative AI engine automatically generates test scripts and data automatically; helping organizations streamline testing processes while speeding time-to-market.
- In 2022,ย Functionize enhanced its generative testing capabilities with the addition of an enhanced machine learning model that enables more accurate and intelligent test case generation, improving testing activities' effectiveness and ultimately software quality.
FAQ
1. What is Regenerative Artificial Intelligence Testing (RAIT)?
A. Generative AI testing refers to using artificial intelligence algorithms to automate and enhance software testing processes by producing test data, test cases, and scripts automatically.
2. How does Generative AI testing improve software quality?
A. Generative AI testing improves software quality by creating diverse and realistic test scenarios, increasing coverage and uncovering defects or vulnerabilities that would go undetected with traditional approaches to testing.
3. What are the advantages of employing generative AI in testing?
A. Use of generative AI in testing can result in faster and more efficient testing processes, improved test coverage, increased defect identification accuracy and shorter time-to-market periods. Furthermore, using this technology enables testers to focus on critical tasks to enhance productivity and cost savings.
4. Are there any ethical considerations associated with testing generative AI?
A. Ethical concerns regarding generative AI testing may arise in relation to its biased or discriminatory data generation. Therefore, it is critical that test data generated is managed and validated thoroughly to ensure fairness and prevent any potential biases during testing process.
5. What challenges arise from using generative AI for testing purposes?
A. Implementing generative AI for testing can present numerous obstacles, including assuring quality and reliability of AI models, closing skill gaps in AI technologies, managing resistance to change and dealing with data privacy and security concerns.
6. Can Generative AI Testing Integrate With Existing Testing Frameworks and Tools?
A. No need to be overwhelmed! Generative AI testing solutions are tailored to seamlessly integrate into existing frameworks and tools, giving organizations an easy way to incorporate these features into their testing workflows.
7. Are AI testing systems suitable for all industries?
A. Generative AI testing can be applied across various industries, such as IT, healthcare, finance and automotive. Because comprehensive and efficient testing processes exist in most sectors, generative AI in testing offers organizations looking to increase software quality a powerful way of improving software quality.
Contact us
Contact Person:ย Mr. Lawrence John
Marketresearch.Biz
Tel:ย +1 (347) 796-4335
Send Email:ย [email protected]
Content has been published via 11press. for more details please contact at [email protected]

The team behind market.us, marketresearch.biz, market.biz and more. Our purpose is to keep our customers ahead of the game with regard to the markets. They may fluctuate up or down, but we will help you to stay ahead of the curve in these market fluctuations. Our consistent growth and ability to deliver in-depth analyses and market insight has engaged genuine market players. They have faith in us to offer the data and information they require to make balanced and decisive marketing decisions.