Generative AI in the Enterprise Market size is expected to be worth around USD 37,656 Mn By 2032
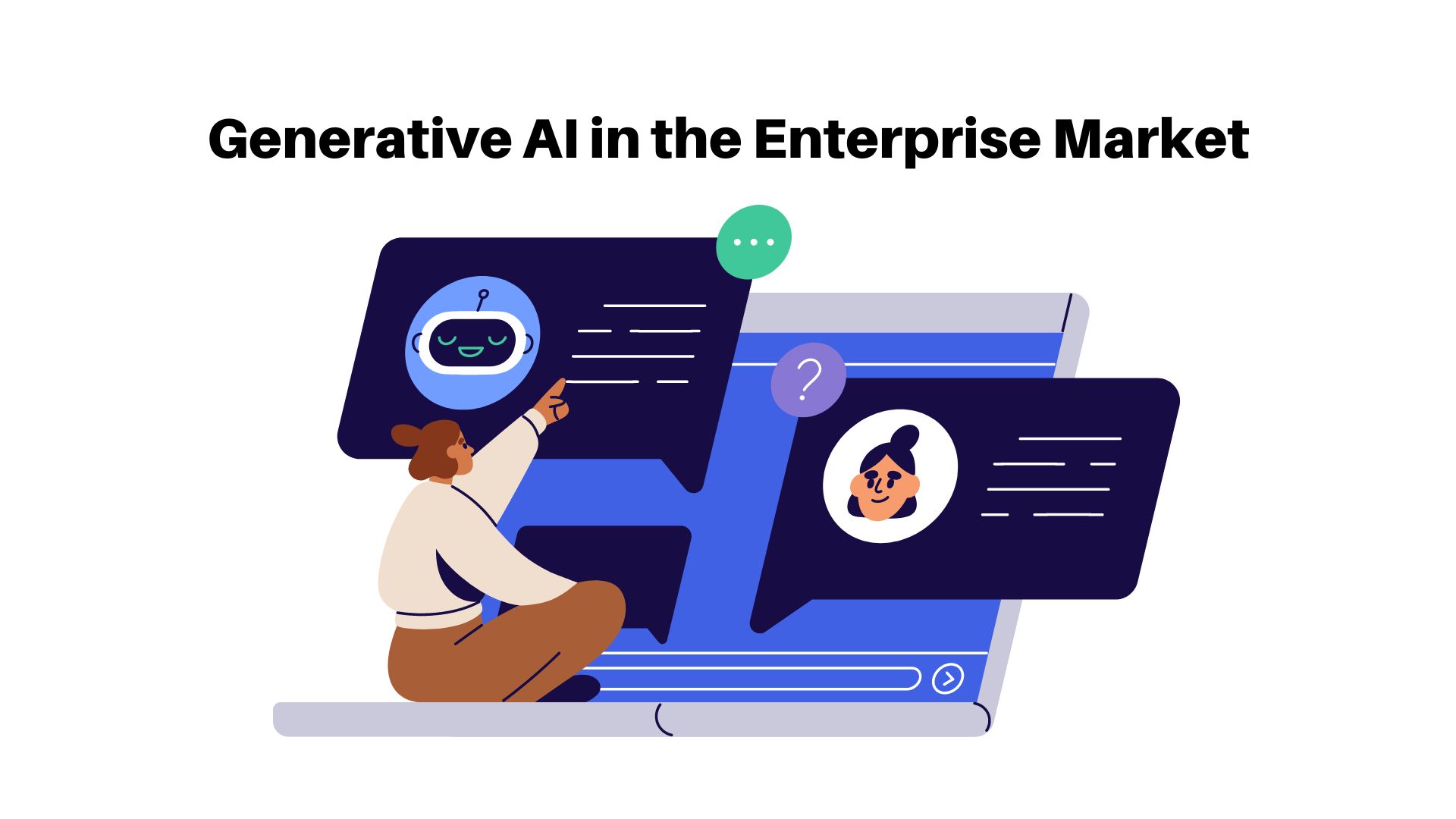
Page Contents
Market Overview
Published Via 11Press : Generative AI in the Enterprise Market size is expected to be worth around USD 37,656 Mn by 2032 from USD 1,476 Mn in 2022, growing at a CAGR of 39.4% during the forecast period from 2022 to 2032.
Generative AI, also known as Generative Adversarial Networks (GANs), has emerged as an innovative technology with significant implications for enterprise markets. Combining machine learning and deep neural networks to generate new, original content from existing data patterns. Generative AI has found application across various industries such as marketing, design, finance and healthcare.
One area where generative AI is making waves is marketing. Companies are turning to this technology to craft personalized and engaging content for their customers, creating ads, product recommendations and social media posts tailored specifically to individual tastes that not only engage but increase conversion rates as well as drive revenue growth.
Generative AI is revolutionizing the creative process within the design industry, offering designers new possibilities when exploring ideas. They can simply input specific parameters and constraints, while AI generates numerous design options allowing rapid prototyping and testing out of different concepts – saving both time and enhancing creativity – from product designs to architectural concepts; Generative AI is pushing innovation limits across enterprise sectors.
Finance is another area where generative AI has seen tremendous advancement. The technology can analyze vast amounts of financial data, identify patterns, and generate predictive models for stock prices, market trends and risk analysis – helping financial institutions make informed investment decisions, manage portfolios more efficiently, mitigate risks more effectively and mitigate their own liabilities. Furthermore, algorithmic trading platforms use Generative AI algorithms to identify profitable trading strategies using historical market information.
Generative AI is revolutionizing healthcare through diagnostics, drug discovery, and personalized medicine. Medical professionals are using this technology to analyze patient data, detect patterns, generate predictive models for disease diagnosis and treatment recommendations, simulate drug interactions to accelerate drug discovery process as well as customize treatment plans based on an individual's genetic makeup to enhance patient outcomes.
Though generative AI presents great potential, there are challenges and ethical considerations associated with using it for enterprise market. Privacy and security issues arise when handling large amounts of sensitive customer or patient data. Furthermore, transparency and interpretability must ensure generated content matches up with ethical standards without reinforcing biases present in training data.
Request Sample Copy of Generative AI in the Enterprise Market Report at: https://marketresearch.biz/report/generative-ai-in-the-enterprise-market/request-sample
Key Takeaways
- Generative AI is revolutionizing marketing by producing personalized and engaging content based on customer data.
- Designers can utilize generative AI to quickly explore various design possibilities, saving both time and fostering creativity.
- Financially speaking, generative AI is providing more informed investment decisions and risk management by using predictive modeling and algorithmic trading systems.
- Healthcare has seen great results from applying generative AI to diagnostics, drug discovery and personalized medicine – ultimately improving patient outcomes.
- Concerns related to privacy and security must be considered when handling sensitive data in generative AI applications.
- Transparency and interpretability are fundamental to ethical deployment of generative AI and mitigating bias within its generated content.
- Generative AI holds great promise to drive revenue growth and boost conversion rates within the enterprise market.
- Enterprise markets must embrace generative AI while carefully considering its ethical implications and ensuring responsible deployment for maximum benefit.
Regional Snapshot
- North America and, particularly the US, has been at the forefront of generative AI adoption within enterprise markets. Major tech hubs like Silicon Valley have witnessed substantial investments and advancements in this space; companies from sectors like finance, healthcare and e-commerce use generative AI to drive innovation, improve customer experiences and gain a competitive advantage.
- Europe has taken to AI-powered enterprise applications with great enthusiasm, particularly the United Kingdom, Germany, and France where it has seen phenomenal adoption growth. European enterprises use generative AI for marketing campaigns, design automation, financial analysis, healthcare applications as well as ethical considerations and regulatory frameworks that ensure safe deployment.
- Asia Pacific region is witnessing an explosive increase in generative AI's adoption in enterprise markets, particularly across China, Japan, and South Korea. Chinese companies in particular are taking the lead when it comes to adopting and using this form of technology for personalized marketing, design optimization, and healthcare diagnostics – thanks to its strong technological infrastructure and vast consumer base – the growth is unprecedented here.
- Latin America is experiencing a growing interest in Generative Artificial Intelligence for enterprise use. Countries like Brazil and Mexico have witnessed startups and enterprises using Generative AI for marketing strategies, content generation, financial analysis and other purposes. Latin America's growing digital landscape and tech ecosystem provides an ideal setting for adopting Generative AI solutions.
- Although generative AI technology remains in its infancy in the Middle East and African enterprise markets, interest has grown in its potential application. Countries like United Arab Emirates and South Africa have begun exploring its use for marketing, design and healthcare purposes – though as its maturity and awareness spreads this adoption will likely increase further across the region.
For any inquiries, Speak to our expert at: https://marketresearch.biz/report/generative-ai-in-the-enterprise-market/#inquiry
Drivers
Advanced-Data Analytics and Insights
Generative AI provides organizations with advanced data analytics to unlock valuable insights from their vast amounts of information. By analyzing patterns and trends, generative AI generates new content – be it targeted marketing campaigns or product designs optimized to customer preferences – that helps companies make informed decisions, enhance customer experiences and identify untapped market opportunities.
Creativity and Innovation Enhance
Generative AI empowers businesses to unlock new frontiers of creativity and innovation. By automating parts of the creative process such as design generation or content production, generative AI frees up designers' and creative professionals' time for more strategic or inventive tasks within an enterprise. Furthermore, this technology serves as a platform for experimentation, iteration and the discovery of novel ideas which fuel enterprise innovation.
Enhancing customer engagement and personalization
Generative AI empowers organizations to offer highly tailored experiences for their customers. By analyzing customer data and preferences, this form of artificial intelligence (AI) enables content, recommendations, and advertisements tailored directly toward individual customers – an experience that increases customer engagement while creating brand loyalty and conversion rates. Generative AI's capacity for personalized and relevant experiences has become one of the primary factors attracting enterprise market organizations to adopt it as well.
Operational Efficiency and Cost Reduction Strategies
Generative AI can significantly streamline business operations and boost operational efficiencies within organizations, streamlining repetitive tasks such as content generation or design iteration and optimizing resource allocation to accelerate workflows, reduce human error and optimize resource allocation – leading to cost savings, resource optimization and reallocation to more strategic initiatives that increase productivity levels.
Restraints
Privacy and Security Concerns with Data
One of the key barriers to the adoption of generative AI in enterprise markets is increased privacy and security concerns. Generative AI systems often need access to large amounts of data in order to generate meaningful outputs, leading to legitimate concerns over its privacy and security. Enterprises must carefully consider data governance mechanisms like encryption or access controls so as to minimize any unauthorized access or data breaches.
Ethical and Legal Considerations
Generative AI systems have the potential to produce content that may be ethically or legally problematic, for example by accidentally producing discriminatory or offensive material, infringing intellectual property rights, or creating deepfake videos for malicious use. Enterprises should develop solid guidelines and frameworks for using generative AI technology responsibly and ethically – this may involve rigorous content moderation measures, meeting regulatory requirements, and having clear user consent mechanisms in place.
Transparency and Explainability Issues Exist
Generative AI models utilizing deep learning techniques may appear opaque; their decision-making processes cannot easily be understood or explained to stakeholders in enterprise settings where transparency is desired. As a result, this lack of transparency may prevent its widespread adoption – as stakeholders require visibility into how and why certain outputs are generated – while developing methods to provide explanations and justifications for AI-generated content may help build trust while aiding regulatory compliance.
Computational Resources and Scalability (CR&S)
Generative AI models, particularly cutting-edge ones, tend to be computationally intensive and demand significant computing resources, creating challenges for enterprises with limited computing capacities or budgetary restrictions. Furthermore, scaling these systems to process large volumes of data in real time requires powerful infrastructure and efficient data processing pipelines; so enterprises must carefully consider whether implementing or maintaining such solutions fits within their computational requirements and scalability criteria.
Opportunities
Marketing and Advertising
Generative AI creates opportunities for targeted marketing campaigns, personalized advertisements and content production. Organizations can utilize this AI technology to analyze customer data, predict preferences and develop engaging materials that reach specific target audiences.
Design and Product Development
Generative AI offers enterprises new possibilities for rapid prototyping, design iteration and optimization. Businesses can employ this form of artificial intelligence to rapidly produce multiple design options and test variations before finding optimal solutions – shortening product development cycles while sparking creativity and innovation.
Finance and Investment
Generative AI presents opportunities for enhanced financial analysis, risk evaluation and investment decision-making. Financial institutions can utilize this technology to monitor market trends, forecast stock prices and optimize portfolios more accurately than ever. Generative AI also empowers enterprises with data-driven financial decisions with greater accuracy and efficiency than ever before.
Healthcare and Medicine
Generative AI offers healthcare practitioners an unprecedented opportunity for improved diagnostics, drug discovery, and personalized medicine. Doctors can utilize generative AI to analyze patient data, detect patterns in it, generate predictive models that predict disease diagnoses or treatment recommendations using this revolutionary technology – and revolutionize healthcare outcomes while creating tailored treatment plans.
Take a look at the PDF sample of this report: https://marketresearch.biz/report/generative-ai-in-the-enterprise-market/request-sample
Challenges
Skill and Expertise Gap
Integrating generative AI solutions into enterprise environments often requires specific knowledge and expertise. From developing, training, and fine-tuning models through machine learning to data science and software engineering – companies may struggle to hire personnel who possess these necessary skill sets for deployment within their organizations, leading to shortages in talent in this emerging field. Addressing this challenge means investing in upskilling programs for existing employees or partnering with external consultants or consulting firms that specialize in this emerging space.
Integration with Existing Systems
Integrating generative AI technology into existing enterprise systems and workflows can be complex and time-consuming, especially since many organizations rely on legacy systems and databases that may not meet the specifications for generative AI models. Ensuring seamless integration, data interoperability, and smooth communication amongst all components is paramount for its successful adoption; successfully overcoming this hurdle requires extensive knowledge of IT infrastructures as well as careful planning in order to minimize disruptions during implementation.
Data Quality and Availability
Generative AI models rely heavily on high-quality, diverse, and representative data in order to produce accurate and valuable outputs. Unfortunately, enterprises may face difficulties related to data quality such as incomplete or inconsistent data sets, bias in training data sets or lack of sufficient labeled training data sets for training. Accessing relevant sources within an enterprise and putting quality control measures in place are essential ways of overcoming this challenge; businesses may invest in data collection, cleaning, and annotation processes to increase the availability of generative AI models.
Considerations of Cost and Return On Investment (ROI)
Implementing generative AI solutions into enterprise markets may involve significant upfront costs, such as infrastructure investments, data collection and acquisition and talent training or acquisition. Organizations should carefully assess the return on investment (ROI) and long-term value generated by this technology for their specific business contexts; quantifying tangible and intangible benefits like improved efficiency, enhanced customer experiences or new revenue streams is vital in justifying investments and allocating resources accordingly.
Market Segmentation
Based on Technology
- Deep Learning
- Generative Adversarial Networks
- Natural Language Processing
- Cloud Computing
- Other Technologies
Based on the Deployment Mode
- Cloud
- On-premises
Based on Application
- Content Generation
- Design & Creativity
- Virtual Assistants & Chatbots
- Data Augmentations
- Other Applications
Key Players
- OpenAI
- NVIDIA Corp.
- IBM Corp.
- Microsoft Corp.
- Google LLC
- Amazon Web Services, Inc.
- Adobe Inc.
- Salesforce, Inc.
- Other Key Players
Report Scope
Report Attribute | Details |
Market size value in 2022 | USD 1,476 Mn |
Revenue Forecast by 2032 | USD 37,656 Mn |
Growth Rate | CAGR Of 39.4% |
Regions Covered | North America, Europe, Asia Pacific, Latin America, and Middle East & Africa, and Rest of the World |
Historical Years | 2017-2022 |
Base Year | 2022 |
Estimated Year | 2023 |
Short-Term Projection Year | 2028 |
Long-Term Projected Year | 2032 |
Request Customization Of The Report: https://marketresearch.biz/report/generative-ai-in-the-enterprise-market/#request-for-customization
Recent Developments
- In June 2020, OpenAI introduced its GPT-3 (Generative Pre-trained Transformer 3) model, an innovative generative AI model. GPT-3 quickly garnered significant acclaim due to its ability to generate human-like text generation; since then it has found widespread applications within various enterprise use cases including content creation, customer service support and language translation.
- In 2021, Google recently unveiled its Language Model for Dialogue Applications (LaMDA), a generative AI model designed to promote natural and engaging dialogues. LaMDA focuses on improving language understanding while producing contextualized responses, making it suitable for enterprise applications like customer service chatbots or virtual assistants.
- In 2019, NVIDIA's GauGAN was released to the enterprise market as an Artificial Neural Network model capable of rapidly turning simple sketches into realistic images. GauGAN quickly garnered interest within industries such as architecture, urban planning and design where professionals require visual representations or concept art quickly.
FAQ
1. What is Generative AI, and how does it differ from other Artificial Intelligence (AI) approaches?
A. Generative AI refers to a subset of artificial intelligence (AI) which specializes in creating original content from existing patterns or samples in input data, such as images, texts or music. This differs from predictive and classification models which are more focused on making predictions or categorizing existing patterns into categories for processing data.
2. How can generative AI benefit enterprises?
A. Generative AI provides enterprises with many benefits. It can automate content production, aid design and creativity processes, optimize workflows, enhance customer experiences, enable personalized recommendations, generate synthetic data for testing purposes and simulate simulations – enhancing efficiency, innovation and competitiveness across various industries.
3. What ethical considerations exist with regard to generative AI for enterprise markets?
A. Generative AI raises ethical considerations due to its potential misuse, including producing deep fake content or biased outputs. Therefore, enterprises should devise guidelines and practices for the ethical use of generative AI which include transparency, accountability, fairness and privacy protection.
4. What are the obstacles involved with implementing generative AI in an enterprise setting?
A. Implementing generative AI into enterprise markets may present several obstacles, including data privacy and security concerns, ethical and legal considerations, lack of transparency and explainability, computational resource requirements, skill and expertise gaps, integration into existing systems, as well as guaranteeing data quality and availability.
5. How can enterprises address data quality concerns related to generative AI?
A. Enterprises need to invest in data collection, cleaning, and annotation processes in order to address data quality challenges. Enterprises should strive for diverse yet representative data while addressing biases and implementing quality control measures. Working closely with domain experts or employing data augmentation techniques may further boost data used for training generative AI models.
6. Are there any regulatory considerations when applying generative AI to enterprise markets?
A. Yes, regulatory considerations play a vital role in adopting generative AI. Depending on their industry and region of operation, enterprises may need to adhere to regulations concerning data privacy, intellectual property rights, content moderation and fairness in AI decision-making. Staying abreast of relevant regulations and putting into effect necessary safeguards is imperative in avoiding legal and compliance issues that arise with adopting this technology.
7. How can enterprises ensure the explainability of AI outputs?
A. Explainability can be challenging due to the complexity of AI models. However, techniques like model introspection, interpretability methods and feature attribution may provide insights into decision-making processes and can aid enterprises in building trust in generated outputs generated by such AI systems. Enterprises should explore and adopt explainability techniques so as to increase transparency and trust in their generated AI systems' outputs.
Contact us
Contact Person:ย Mr. Lawrence John
Marketresearch.Biz
Tel:ย +1 (347) 796-4335
Send Email:ย [email protected]
Content has been published via 11press. for more details please contact at [email protected]

The team behind market.us, marketresearch.biz, market.biz and more. Our purpose is to keep our customers ahead of the game with regard to the markets. They may fluctuate up or down, but we will help you to stay ahead of the curve in these market fluctuations. Our consistent growth and ability to deliver in-depth analyses and market insight has engaged genuine market players. They have faith in us to offer the data and information they require to make balanced and decisive marketing decisions.